- September 7, 2022
- Posted by: IGBAJI UGABI
- Category: Academic Writing Guide
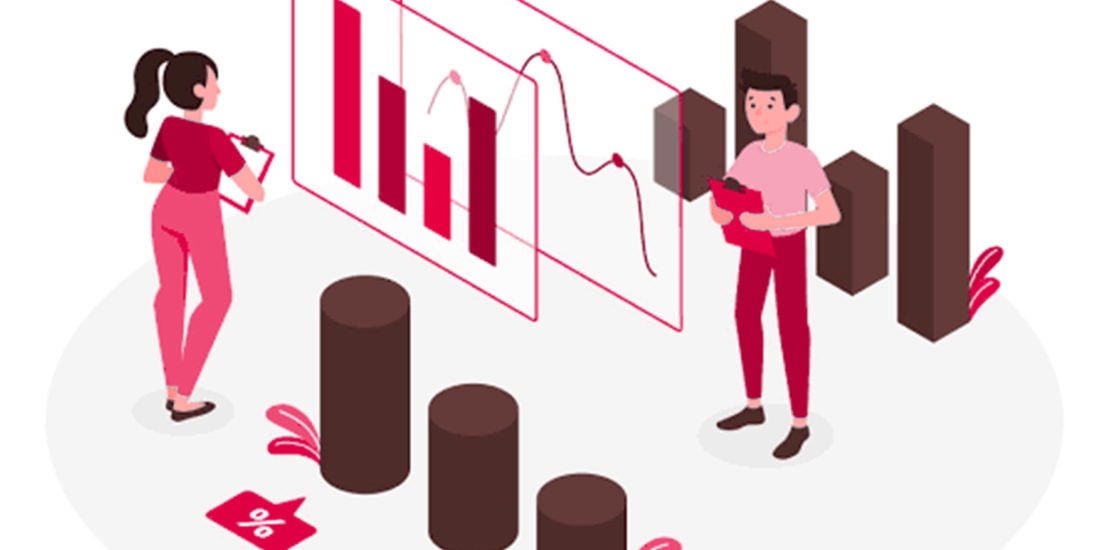
How to do Data Presentation, analysis and Discussion
Introduction
This comes up, usually, in Chapter Four of the research project. This is where the researcher presents the data collected from respondents though not in the raw form. In their raw forms, it is quite difficult to present and analyse data, which is why there is a need for the raw data to be organized and presented in more compact forms. Subjecting the data to tabulation, grouping or even graphic forms, so as to allow for easy handling and analysis, could do this.
In doing this, the chapter sets out on an introductory note often referred to as “Preamble” where the researcher provides useful background information on the respondents’ group (s), their characteristics with respect to their bio-data and the rate of returns of the data gathering instruments.
After this, he moves on to the main theme of his research by presenting necessary data in the form (s) considered most appropriate for the purpose of analysis. If, as an instance, the tabular mode of data presentation was used, the tables should be well titled; each followed by detailed explanation on the data presented. This pattern should be used for each of the tables presented. Also important under data analysis is the Discussion of Results segment.
This comes up, normally, after the entire presentation exercise had been concluded. It is the segment where the researcher gives a more detailed insight into the issues directly relating to the data presentation and analysis. The segment helps to articulate the issues emanating from the data analysis with respect to whatever implications they have on the subject of investigation.
If the study is concerned with hypotheses testing, it is in this segment that the implications of the outcomes of the tests as they relate to the subject of research would be explained. Here also, conclusions on the relationship of the outcomes of the present study with previous ones are drawn; with a view to establishing a link between the outcomes of the present study and those of previous studies as already established under the literature review.
Furthermore, the researcher dedicates a part of this segment to the interpretation of the outcomes of his findings, thereby giving more meaning and sense to the data analysis exercise.
Read also: How to write Research Methodology
The Use of Statistics in Data Analysis
Sulaiman {1997} defined the term statistics as “a branch of applied mathematics, which is employed in analysis of data to facilitate meaningful decision making.” It is also described as the theory and methods of analysis obtained from samples of observation in order to compare data from different empirical observations using hypothesized relationships in order to make meaningful decisions.
Even then, the methods of data analysis depend on the aims and objectives of the study and the nature of the data gathered. It becomes clear from the above, that statistical analysis could be useful for: –
(i) Reducing quantities of data to manageable and understandable
form.
(ii) Aiding decision making
(iii) Summarizing samples from which they are calculated (iv) Aiding reliable references and decisions from the hypothesis
Statistics thus serves as a tool used in collecting organizing, analysing and interpreting data. Generally speaking, statistical methods are categorized into broad classes of Descriptive and Inferential Statistics. Descriptive Statistics are often used to summarise the data collected, while Inferential Statistics are used to determine the generalizability of findings arrived at, through the analysis of a sample, to the larger population.
Note that Descriptive Statistics can be used for both sample and population data but cannot be used to perform inferential tests on population data. This is because the results obtained from the descriptive analysis are definitive enough for the population of interest. The application of either Descriptive or Inferential statistics to a set of data largely depends on the levels or scales of measurement of underlying variables. In all, there are four (4) levels of measurement otherwise known as scale.
Nominal Scale
This is considered as the simplest and the least refined scale of measurement; one whose primary use is to provide a labelling function. A good example of this is the individual’s sex, which can be either male or female. There cannot be any other thing between these two. The Yes or No kinds of questions are also good examples of this. However, it lacks the property of order and magnitude.
Ordinal Scale
This kind of measurement also performs the labelling function apart from its ordering function. This is because it possesses the property of order and magnitude such that two things could be compared in terms of their relative magnitude. A good example of the Ordinal Scale relates to the degree of agreement with a statement such as Strongly Agree, Agree, Disagree, and Strongly Disagree. Using this scale to measure two units, one will be able to determine which is higher or lower and not just that they are not the same.
Interval Scale
This also has the property of order, magnitude and additivity since equal intervals on the scale represent that there is a difference in magnitude. The scale does not possess absolute zero because the zero is arbitrarily set. In addition to its ordering function, this scale can be used to determine the difference between two units. Measuring the temperature of a room in Celsius and Fahrenheit is a good example of this scale.
Ratio Scale
This scale is the highest level of measurement because it has an absolute zero. As a general rule, whatever statistical methods are applicable to variable measured in the nominal scale can be applied to those measured in ordinal and interval/ratio scales. Similarly, those statistical methods applicable to variables measured in ordinal scale can also be applied to those measured in interval/ratio.
There are, however, statistical methods that are applicable to variables measured in interval/ratio that could not be applied to variables measured in the nominal scale. Some examples of the ratio scale include weight, time and speed; thus possessing all the properties of the other scales.
Must Read: Writing Chapter Five of Research Project -Guide to Summary, Conclusion, and Recommendation
In data analysis, there are procedures and tools to be employed depending on the type of research as well as the nature of the data to be analysed. Regardless of the instruments/methods used in data collection, and whether the data is from a sample or population, the first step in data analysis is to describe the collected data. To do this, however, the data should be summarized either using a frequency table or chart. These two are veritable tools for presenting and communicating data in such writings as technical reports and journal articles.
The Frequency Table
There is no doubt that with the Frequency Table, the researcher can display the number of cases, which have each of the attributes of a given variable. It also serves to display both qualitative and quantitative data. When confronted with the number of attributes or categories of a variable that is too large, the Frequency Table adopts the grouped data approach by combining the attributes into classes.
E.g. with Age as a variable, the Frequency Table may present data
as: –
20-24 25-29 30-34 35-39 40-44
The Charts
Just like the Frequency Tables, there are also Charts, which serve similar purposes. The two most commonly used Charts are the Pie and Bar Charts. That is, both could be used to present data summaries and also used to interpret and convey the message more quickly, concisely and clearly than frequency tables. Their great limitation however lies in the fact that they hardly cope in situations where the attributes of a variable to display are too many, especially when these are more than nine.
This is particularly so for Pie Charts which are quite useful in providing vivid picture of data but only in showing the distribution of variables with single responses. Thus, they are inappropriate tools for variables associated with multiple responses from the units of the study. Also, while they are most applicable for qualitative data, Pie Charts also serve to display quantitative data particularly those whose number of attributes or categories is not more than five.
As for the Bar Charts, they serve for qualitative data in particular, irrespective of the nature of the responses to the variables, either single or multiple. Since Bar Charts make it easier to compare the categories of a variable, they are more suitable for displaying data with more than five categories. They also serve to display quantitative data, particularly, the variable presented in a discrete fashion. However, Histogram remains the more appropriate tool for displaying continuous variables.
Recommended: Thesis and Dissertation -What are the Differences?
Measures of Central Tendency
This is another approach to describing a set of data, considered useful in determining a typical attribute/value of a variable. The measure is also useful in comparing the performances of two or more groups or the performance of a group over two or more periods of time. The Mean, Mode and Median are the three most common Measures of Central Tendency.
The Mean
The Mean is the arithmetic average of a set of data usually applicable to quantitative data. To obtain the Mean, sum up all the scores in a set of data to be divided by the number of scores. With the distribution of the variable that is skewed, however, the Median will better represent the distribution, as extreme values tend to increase or decrease the Mean.
The Median
The Median is considered as the middle value in a set of data when all the values are arranged in order of magnitude. In other words, the Median tends to show the grouping together of scores around a central point, dividing a set of data into two main parts. In short, the middle scores between the upper half and the lower half is the Median. Although the Median is most appropriate for Ordinal Data, it is also applicable to Ordinal, Interval and Ratio Data.
The Mode
Meanwhile, the score, which has the largest frequency in a set of data, is referred to as the Mode. It refers to the most common attributes or value of a variable in which case it is possible for a set of data to have more than one Mode. Although most appropriate for Nominal Data, the Mode is also applicable to all types of data.
Measures of Variability
This is also known as the Measures of Dispersion in which a measure of variation or dispersion is calculated primarily to determine the homogeneity of a set of data. There are separate measures of variation for qualitative and quantitative data. For quantitative data, measures of variation include: –
(i) The Range
(ii) Standard Deviation
(iii) Variance or the Square of the Standard Deviation (iv) Coefficient of Variation
The Range
This refers to the difference between the highest and lowest attribute or value. Its primary objective is to give the researcher an idea of the data spread to determine the range for a grouped data, minus the highest limit from the lowest limit. Thus, the range is solely based on the two extreme values and fails to recognise how the data are actually distributed between these two values. Hence, the desirability of Standard Deviation to offset this inadequacy.
Standard Deviation
This is defined as the distance or the average deviation of all values from the Mean. The difference between each Score and the Mean is the Deviation Scores from the Mean. The bigger the Deviation, the more variable the set of Scores. The Standard Deviation is obtained by taking the square of the average of these deviations and divided by the number of Scores. Thus, it is an indication of the typical deviation of the values from the Mean. If the Standard Deviation is small, the group is considered homogeneous whereas a large Standard Deviation is an indication of a heterogeneous group.
Variance
This refers simply to the square of the Standard Deviation, obtained by subtracting each observation from the Mean (x), squaring the resulting difference (Xi -X) to eliminate negative signs of Deviation. They are added up to give the Sum of Squares (Xi-X) and finally dividing it by the number of observation ‘n’.
Coefficient of Variation
This is the Ratio of a distribution’s Standard Deviation expressed to its Mean, multiplied by 100, and is independent of the unit of measurement. Coefficient of Variation is employed when comparing the Variability of two sets of data particularly when they are expressed in different units of measurement.
We Recommend: Project Writing tips
Statistical Hypothesis Testing
Unlike the general discussion on hypotheses as earlier on presented, the topic is being re-visited here (under data analysis), with particular reference to Inferential Statistics. By Inferential Statistics, we refer to drawing conclusions regarding the Population of the Study based on the information obtained from the Sample.
It means that this kind of Statistics will not be relevant in situations such as when one is working with Population Data and when one is not interested in making a general statement about the Population. At the centre of Inferential Statistics is the concept of Hypothesis Testing. This refers to the process whereby the research infers from a sample whether or not to accept a statement about the Population; where the statement itself is the Hypothesis.
Also Read: Research terms and their meaning
Hypotheses are stated either in the Null or Alternative forms for the researcher to validate; even though the Null Hypothesis remains the more commonly used of the two. As a matter of fact, it is always the Null Hypothesis that gets tested and it is mainly on the condition that it is rejected that one can accept the Alternative Hypotheses.
When testing Hypotheses, the maximum probability with which one may be willing to reject the Null Hypothesis is referred to as the Level of Significance. It is common practice to use an alpha level of 0.05 or 0.01; meaning that there are 5 or 1 of 100 chances of committing Type 1 Error. When the Reject Decision has been made at 0.05 level, it means that the outcome of the experiment is statistically significant at the 0.05% level.
The procedure, which enables one to decide whether to Reject or Accept Hypotheses or to determine whether observed Samples differ significantly from expected results is differently referred to as Test of Significance, Rules of Decision, or Test of Hypothesis. Thus, if against the assumption that a particular hypothesis is true, we find results observed in a random sample differ markedly from those under the hypothesis, we then conclude that the difference is Significant. On this basis, we can Reject the Null Hypothesis. Errors are sometimes made in Hypothesis testing and these have been categorized into: –
C a) Type 1 Error Cb) Type 11 Error
In a situation where we Reject the Null Hypothesis when, in fact, we should Accept it, it is said that we have committed a Type 1 Error of decision or judgement. On the other hand, if we Accept the Null Hypothesis when we should, indeed, reject it, we are said to have committed Type 11 Error. Such errors usually lead to wrong decisions.
To have a good Test of Hypothesis, there must a design to minimise these errors of decision. A sure way of doing this is to increase our sample size, since the larger the Sample Size, the less the possible errors. Some of the several kinds of Inferential Tests often employed in the analyses of data include: –
(a) T-Test
(b) Analysis of Variance Cc) Chi-Square
(d) Correlation and Regression Analyses
T-Test
This is normally used to compare the Means of two groups of data; which means that the data being compared should be quantitative. These two groups of data may be for two independent samples or maybe for the same sample with the data collected at two different periods {i.e. paired samples}. If, based on the observed p-value, it is decided that the two groups are different, then, one should be able to state which group has the larger Mean.
Analysis of Variance
This Test, commonly referred to as ANOVA, is normally used to examine the effects of qualitative independent variables on a quantitative dependent variable. The One-way ANOVA is its simplest form and is used for comparing the Means for several groups. If, in the end, the Null Hypothesis is Accepted, it indicates that the Means for all the groups are the same.
On the other hand, a Rejected Null Hypothesis indicates that not all the Means are the same even as it does not mean that they are all different. To ascertain which pairs of means are different, it becomes necessary to conduct a multiple comparison test.
Chi-Square
This kind of Test is often used to determine the existence of a relationship between two qualitative variables. Before applying the Test at all, a Contingency Table {Cross-tabulation} is usually formed to study the patterns of frequencies in the Table. If, at the end, the Null Hypothesis is Rejected, it means that there is a relationship between the two variables. It is after this that measures are used to determine the strength of the relationship
Correlation and Regression Analyses
These are used to study the existing relationships among quantitative variables; especially those between two quantitative variables. In particular, Correlation Analysis measures the strength of the relationship between the two variables, while Repression Analysis develops an equation that enables one to predict the value of the Dependent Variable for different values of the Independent Variable.
These two methods are commonly used either as Descriptive or Inferential procedures. As a Descriptive procedure, a Correlation Coefficient is calculated to determine the strength of the relationship between two variables. As an Inferential procedure, Correlation Analysis determines whether the observed correlation between the variables as determined from the sample can be generalized to the population.
The procedure requires that the p-value is calculated and used to Accept or Reject the Null Hypothesis. If the Null Hypothesis is accepted {i.e. there is no correlation between the two variables in the population}, there is no need to obtain a Regression Equation, as it cannot be used to predict the value of the dependent variable.
REFERENCE
Sulaiman, S. N. {1997} Statistics & Analytical Methods for Researchers. Kaduna. NDA Computer Centre.
Read: Data Presentation Technique -Choosing Data Analysis and 3 Data Presentation Techniques
what are the essential features of presentation and analysis of data collected in research